How AI in DAM can power your digital asset management
June 29, 2023 •Ricky Patten
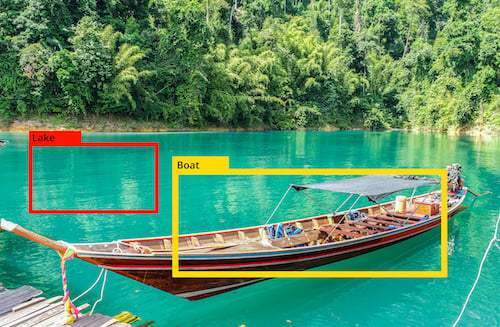
AI and digital asset management has always been a topic of interest for me, one that I've paid particular interest in in the last 20+ years. My first AI project was in the 90s for Sydney University Language Department, where the professor I was working with wanted a computerised analysis of language roots and derivations.
In the next few years, as I started to work extensively with DAM, I noticed that all the customers had the same simple question:
How can I avoid or reduce the time it takes to enter all the metadata?
Is artificial intelligence (AI) in DAM the answer to this question?
Over the last decade, AI developments have made positive impact on our daily working lives. However, the introduction of ChatGPT by OpenAI at the end of 2022 has placed artificial intelligence on a completely different level, soon (if not already) to forever change how we communicate, work, learn and connect.
AI in DAM has been around for a while and, coupled with other technologies, has been helping marketing teams to reduce manual tasks, speed up content creation and better manage creative workflows.
Although, often marketing and creative teams are unaware of these AI capabilities within their DAM solution.
AI to power your DAM
Since I started working with Canto DAM many years ago I have been fully aware of the extensive integration with AI that Canto has implemented in their solution. It was not until recently when I embarked on rounding up all of Canto’s AI capabilities into one document, that I realised how extensive Canto’s AI capabilities really are.
If you're evaluating digital asset management systems, I suggest you download and read my eBook: DAM and AI: Adopting Artificial Intelligence (AI) as the Cornerstone of your DAM Project, for an extensive discussion on how best to use AI capabilities within your DAM, how to correctly set your business objectives, align your DAM team for the adoption of AI and more.
The eBook will give you a good overview of how AI can be used to improve your DAM process, and more importantly, apply that to your own core business needs. What matters the most to you is more important than what matters the most for almost everyone else! Towards the end of the eBook I discuss in length a process to evaluate the relevant importance of investing resources with your DAM content experts versus AI assistance. This will give you a checklist of what AI capabilities will best suit your circumstances.
Below, with the help of Canto DAM, I will introduce you to the AI tools in DAM that can make your working life easier. First to note, Canto uses Amazon AWS technology stack and to date all the AI capabilities Canto offers are based upon Amazon’s AI technology (Rekognition, Textract and Transcribe). Amazon is considered one of the top four AI providers. Many of the below AI capabilities are included in the Canto DAM base solution free of charge.
Smart Tags - i.e. generic image recognition
Any organisation that produces a large amount of digital assets should have a proper tagging and metadata entry process in place, without that the stored assets become unusable and of no value, as they can't be found. Manual tagging can be time-consuming and inefficient. But are Smart Tags the answer for this in your case?
Smart Tags (called Labels by Amazon) uses AI to identify objects, colours, scenes, activities, image quality, and more in your images and videos, and applies the relevant tags to the assets.
Are Smart Tags accurate? Considering that the AI is essentially working without any previous context determined, then YES Smart Tags are quite accurate. As in all machine learning technology, image recognition accuracy is learned. The number of available, detectable tags (or Labels as they're called by Amazon Rekognition) is constantly growing and new labels are added for objects to be recognised.
Do Smart Tags replace manual tagging? Yes and No. Yes, Smart Tags will apply relevant generic descriptions of objects in your image. No, if you're looking for tags relevant to your business, such as a particular event or tags specific to your organisation.
Smart Tags will not be able to provide tags relevant to your business, for such tags the metadata will need to be applied manually. Take a look at the two examples below. First, a generic image of a sea turtle in its environment successfully tagged with Smart Tags. The second image is of a particular meeting at your office tagged with generic Smart Tags. Note no specific tags of this meeting were applied, as they are not known to the AI tool.
You can improve the number of inaccuracies or random non meaningful tags provided by changing the default settings, i.e. increasing confidence and reducing the number of tags provided. Our current recommendation for Canto DAM is to adjust the confidence level of Smart Tags from the default of 80% to 95% confidence. The higher confidence level means that the AI tags are very relevant.
In comparison, a manually entered tag would have 100% confidence.
You can also change the number of Smart Tags generated. The default is 30 tags, which is quite a lot of tags, often perceived as being random by some organisations. Our current recommendation is to decrease the default tags generated to 5 tags. With 95% confidence and 5 tags generated the result would be 5 relevant tags applied automatically to your assets and more time for your marketing team to focus on other things.
For generic image recognition to have greater benefit, I have recommended to Canto to add an automated filtering to the Smart Tags functionality that would filter the proffered tags to include only those tags that have been entered manually and/or limited to a predefined list. If Canto were to adopt this idea, then we would have a wonderful Smart Tag feature that matched your specific core business needs.
Smart Tags won't work for all organisations, however together with other AI tools such as Facial Recognition and Text Recognition, described later in this article, you can create a powerful, useful and efficient tagging experience. To learn about custom auto-tagging please download my eBook where I explain in detail the possibilities of custom AI projects.
Get Smart Tags for images free of charge with your Canto DAM subscription. Smart Tags for Video is an extra charge.
Facial Recognition
Facial Recognition is a high value and very useful capability that we see used frequently with great benefit.
Described by Amazon as Face Compare and Search and Celebrity Recognition.
Facial Recognition is very accurate and can be a real time saver in DAM. Great to be used to identify people from your organisation, students at your school, people at an event, etc. For it to work, you are required to identify and tag the person in a photo with their name, next this person will be identified throughout your entire DAM library by the Facial Recognition tool.
Facial Recognition works equally well in images and in videos. Take a look at best practices to achieve desired results in this Facial Recognition in Canto Best Practices blog post.
Facial Recognition is especially useful if someone withdraws their permission for their images to be used. Their photos can easily be found and further tagged with "restricted", "do not use" or other status.
How accurate is Amazon's AI for facial recognition?
I have to say I am always very surprised by how good Amazon's facial recognition really is. In all my experiences and testing, we acquire stock photos with consistent talent spread across them, and in talking with our customers, the facial recognition feature works with very few inaccuracies.
Just about every organisation that I see using DAM have a very high proportion of content that includes people. Sometimes it’s 80% plus of all content have people’s faces that are known to the organisation. In many cases, especially where events, students, employees, etc are the focus of the DAM project the only content that can not be identified by the names of the people included is very limited. For example, a school might have 90%+ of their content with students, teachers, staff included and only a very limited range of content which shows buildings and locations without any people.
With facial recognition a significant, suggest between 30-80%, of all time spent on entering metadata can be automated quite accurately and very simply.
Let's take a look at the same image from above now with faces recognised, making the metadata more relevant to your organisation.
Celebrity recognition
This is the other side of face recognition and as far as I have tested works just as effectively as manually entered face recognition for your people. My experience is that with a few exceptions most of the content our customers are working on does not include any celebrities. There are some exceptions and I have had reported a few surprising instances of celebrities being recognised out of context, i.e. within the audience of a school event, but to date have not seen a primary business usage of recognising celebrities.
Some important exceptions to this have arisen lately mainly in conjunction with sporting or activity based organisations. Many events that are captured in such organisations content have high profile celebrities attending typically to support the events. This is a great case of catching those celebrities as they enjoy a footy match!
Get Facial Recognition for images free of charge with your Canto DAM subscription. Facial Recognition for Videos comes at an extra charge.
Hex Colour Code Recognition
A very useful AI capability and has the potential to be used by more DAM users. Hex Colour Code Recognition can only be used for images. The draw back is - only a small percentage of consumers searching a DAM for content, will understand what a Hex Color Code is and will want to filter content by specific Hex Color Codes. Those consumers that fit into this profile will find Hex Color Codes as absolute GOLD (sorry about the pun!).
There is a bit of setup to implement this feature, but once done - any still image, i.e. photo, illustration etc. will be analysed for the Hex Colours included and tagged appropriately. The Consumer’s interface is then configured to provide for Filtering based on Hex Colours. In the world of brand management, design and, in particular, for agencies this is very useful, as company’s logos and overall branding is often based upon the usage of very distinct colours.
A great example of practical usage of Hex Color Recognition was suggested at a recent demo for a prospective customer with a twenty year backlog of content to catch up with. It was explained that over this period the organisation has gone through many re-brandings, each time changing the primary company colours. Using Hex Color Recognition, this backlog of content can be quite accurately related to various time periods where one brand or another was prevalent.
Get Hex Colour Code Recognition free of charge with Canto DAM subscription.
Transcription
For some organisations Video Transcribe can provide a reliable cost effective basis for accurately tagging their entire video library.
Transcription is another high value capability that is very well utilised. How it works - the output from Amazon Transcribe is stored in the Video Captions field, which can be viewed and edited to correct any inaccuracies. All of the text in the Video Captions field is automatically searchable, which is amazing! For example, if the word “London” is mentioned in a video, then when a Consumer searches on London, the video will appear in the search results. The Video Captions field is also used to provide Closed Captions during video playback, increasing the accessibility of content considerably.
Transcription is perhaps one of the best ways to automate metadata entry for video content, as long as your videos have human voice. Note, transcription also works for songs and other non conversational human speech.
Video presents its own high level of complexity when considered within a metadata entry context. Simply put, it takes much longer to watch through a video and collect applicable tagging than it does to look at a still image. This is why Transcription is so compelling for organisations to utilise, for automated metadata entry of video content.
Video Transcription is available for an extra cost in Canto DAM.
Text Detection
As described by Amazon, Text Detection Extracts skewed and distorted text from images and videos of street signs, social media posts, and product packaging.
I think it is one of the most under-rated key features in a DAM. I personally find that Text Detection has a wide range of potential uses and is unfortunately under-utilised by our customer base.
Canto has implemented Text Detection for images only at the time of writing.
A very good example is the following image:
The Text Recognition feature detects words exactly how they are showed in an image and makes that text searchable. From the above example this includes street numbers, hand written signs, and phone numbers! Very simple and with a high level of accuracy.
We have seen this tested with name tags on students uniforms, building names, advertising and more. All working as expected, providing a high level of findability based upon the environment in which an image has been taken.
The possibilities are endless, as an example, in an asset management solution for the construction sector, industrial equipment could be recognised based upon serial numbers or number plates. Using Text Detection and date image taken metadata along with the GPS co-ordinates it would be possible to automatically identify when valuable equipment has been used in various locations and the last known location of the kit.
Why we don’t see Text Recognition being used as often as it should be? I’m a bit of a loss to say why but I do think with the plethora of other AI options, in particular Facial Recognition which is a big winner, Text Recognition is simply overlooked. I’m taking this on as something that databasics can improve on, with more attention during the onboarding process and proactively finding usages for such inbuilt features to benefit our customers.
Get Text Recognition free of charge with Canto DAM subscription.
Optical Character Recognition (OCR)
The OCR feature in Canto is using Amazon’s Textract feature. This is described as a machine learning (ML) service that automatically extracts text, handwriting, and data from scanned documents.
OCR works on pdf document only. The OCR feature is effectively performing what was previously implemented in document scanners as OCR. The process to recognise the optical characters has been moved from the scanner technology to Amazon.
This is a feature that is used by our customers extensively. It is commonly expected by most users that they will be able to search on their PDF documents by the embedded text. As such OCR meets a user expectation and gets a high level of usage.
Get OCR free of charge with Canto DAM subscription.
Summary
The most highly regarded AI capability commonly available within Canto is Facial Recognition, and for good reasons. Faces are everywhere and form a majority of searchable content for most users. When implemented well, Facial Recognition works easily and provides for instant findability across both images and video.
Transcription for video and OCR for PDFs are used commonly and are highly valuable by our customers. The spoken and written word is common throughout most digital assets and there is a high level of expectancy that this content will be available during the search process.
Other forms of AI are also available within Canto and get utilised to various levels depending upon applicability to the content being managed, user’s expectations and front of mind awareness. Perhaps better user interfaces, and more informed onboarding processes will increase the uptake of more forms of AI capabilities within Canto.